Elderly Acoustic Model for Large Vocabulary Continuous Speech Recognition
全文
(2) T冶ble 3: HMM configuration parameters. HMM model monophone triphone PTM. I I I. state. 43x3 2500. I. 2500. Table 6:. mixture. number of Gaussian. 16. 43x3x16 2500x 16. 16 64. JNAS speaker word correct rates by JNAS. acoustic models. 43x 64. Table 4: Acoustic feature window length window interval parameter CMS. I. 25msec hamming. I I. applied by a whole sentence utterance. I. 100 95 90 "IIt 苦85 • �U 80 375 70 65 60. 10msec MFCC+ムMFCC+ムPow(total 25 dim.). built from a newspaper, and the lexicon size is 20k. Th巴 test set contains 200 sentences uttered by 46 elderly test. speakers, who are different from 301 training speakers. 3.2. Acoustic model The elderly acoustic models are trained from the elderly speech database. The JNAS acoustic models are trained from the JNAS speech database. Three kinds of acoustic models (monophone, PTM, and triphone) are trained. as. 一←elderly-male. 一←・Id・rly-female. JNAS-male ー . �. . JNAS-f,・male . . E>'. 5. the elderly acoustic models and the JNAS acoustic mod els. The HMM configuration parameters for each acous. 9. 13 test sp・aker. 17. 21. Figure 1: Each speaker's word correct rates for gender. tic model are listed in Table 3. Each acoustic model is. dependent PTM models.. left-to-right three state HMMs of 43 phones. The PTM. (Phonetic Tied Mixture) model is made from monophone. models with 64 mixture components per HMM state. by the JNAS acoustic models. From the results in Ta. by assigning different mixture weights according to the. shared states of triphones[2].. ble 6 and Table 5, there is 6.6% degradation for elderly. To deal with the differ. speakers with the JNAS monophone model (GI), 6.1%. ences between genders, we train both gender-independent. degradation with the PTM model (GI), and 8.4% degra. (GI) models and gender-dependent (GD) models for each. dation with the triphone model (GI).. HMM. Acoustic feature parameters are shown in Table. Figure 1 shows word correct rates for each test. 4 3.3.. speaker by the PTM gender-dependent models1. These results show that elderly speech recognition is especially. Evaluation of acoustic model. di伍cult for male.. results. by JNAS acoustic models. To investigate the improvement by the elderly acoustic model, the elderly speech is recognized by the elderly acoustic model and the JNAS acoustic model.. 3.3.3. Effect of database size. Table 5. To investigate the effect of database size, we train the “JNAS+Elderly acoustic models" from the both. shows word correct rates for elderly test speakers using each model. The word correct rates by the JNAS acous. databases of the elderly one and the JNAS one. Table 7 shows r巴cognition results using the JNAS+Elderly acous. tic models are shown in the parenthesis. In Table 5, the results are shown for male average, female average and. tic models. The acoustic models are gender-independent. total average in GI and GD models of the monophone, the PTM and the triphone HMM models.. models, which are trained through the same procedure as the elderly acoustic models and the JNAS acoustic mod. Compared. with JNAS models, the elderly GI acoustic model attains. els. In Table 7, the results are shown separately in elderly speakers and JNAS speakers. Their word accuracy rates. 4.3% improvement for the monophone model, 2.9% im provement for the PTM model, and 5.0% improvement. are shown in the parenthesis.. for the triphone model. T hese results show that the el. Compared with the results in Table 6 and Table 5,. derly acoustic models are much better than usual adult. there are 2.1 % degradation for JNAS speakers and 0.2% degradation for elderly speakers with the monophone. acoustic models for elderly speech. model.. 3.3.2. Comparison' with usua/ adult speech recogπition. are same. With triphone model, there is 0.1 % degrada-. We compare the recognition rate of usual adult speech Table 6 shows recognition. lThe results for test speakers are ordered according to their. results (word correct rates) for the JNAS test speakers. word correcもrates.. 1658 -. With PTM model, there is l.1 % degradation For elderly speakers, both results. for JNAS speakers.. rate. with that of elderly speech.. Elderly female result using elderly. acoustic model is almost七he same as the JNAS female. 3.3.1. E/der/y acoustic model. 164ー.
(3) Table 5: Elderly speaker word COTTect rates(%) by elderlY acoustic model.. 0. is rates by JNAS aco凶tic model.. Table 7: JNAS+Elderly acoustic model results, word cor. Table 9:. rect, and word accuracy shown by. HMM sufficient statistics using JNAS+Elderly acoustic. ‘monophone 81.5(78.0). I. 0. Speaker adaptation by speaker selection and. models,. PTlV. 訂司有. 0). monophone. (N=20). PTM. (N=40). Table 8: Elderly speaker's adaptation results by MLLR.. 4.2.. Speaker aclaptation by speaker selection and. sufficient statistics When the elderly speakers use a speaker adaptation method such as MLLR, they have to utter a lot of sen tences, lt is a hard workjob especially for elderly persons. We test an unsupervised adaptation method, which use. tion for both JNAS speakers and elderly speakers. These. the sufficient HMM statistics from selected speakers[6].. results shows, elderly acoustic models and usual adult. ln this method, only one arbitrary utterance is required,. models are required for better speech recognition.. 4.. 4.2.1. Adaptation effect. Speaker adaptation. The sufficient HMM statistics are calculated for each 561 speakers who was used in the JNAS+Elderly HMM.. In this section, we test two adaptation methods for el derly speech recognition.. For selecting a subset of these sufficient HMM statis. From the result in 3.3, it is. tics, speaker GMM models consisting of the 64-Gaussian. more difficult than usual adult speech to achieve high. mixture model, which is a phone-independent one-state. recognition rate in elderly speech recognition. To achieve better recognition results, the speaker adaptation meth ods have been widely used.. HMM, are used. The GMM acoustic likelihoods for one sentence utterance uttered by test speakers are used. The adapted HMM was synth巴sized from the top N-nearest speaker's HMM sufficient statistics. The numbers of N. 4.1. MLLR speech aclaptation. are 20,40,60,80, and 100 in the experiments. The best results are shown in Table 9,. MLLR[5] is a popular scheme and it has been widely used. In this experiments, the adaptation of HMM pa rameters is done for means of Gaussian mixtures. The. tial speaker-independent model's results by monophone. With PTM model, the improvement rate is 1.5%. These. utterances used by MLLR are different from the HMM training set.. In the Table 9, the el. derly test speaker achieve 2.9% better results than ini. results shows that this adaptation method attains bet. We investigate the effect of initial HMM. models for MLLR elderly speaker adaptation. We adopt the JNAS usual adult �odel and the elderly acoustic �odel as initial baseline HMM phoneme models. The MLLR adaptation is done for ea�h speaker.. ter adaptation effect than the supervised MLLR by 10 sentence utterances.. The experiment results are shown in Table 8. When �O sentences are used, the elderly initial model is 1.3% ' ?etter than the JNAS initial mod�l for monophone, 1.9% better for PTM. W hen 50 sentences are u;ed. there is. We tested the initial adaptation model effects for the. 4,2.2. Effect 01 initial adaptation model elderly acoustic model and the JNAS acoustic model. The adapted HMMs are synthesized from a subset of 301 HMM sufficient statistics for the elderly test speak. 叫m ost no difference of both models for monophone, and the el derly acoustic model is 1.3% better tha� the jNAS. ers, These HMM sufficient statistics are calculated using the elderly acoustic model.. acoustic model for PTM. These results show that the l í tial bas eline model is important for MLLR, and the . elderly aco ustic model is us�ful for speech adaptation of elderly sp eakers.. As for the number of se. lected speakers, it is 20 for the monophone and 40 for. �. the PTM, respectively. For the JNAS test speakers, we use the JNAS acoustic model as the initial model and 260 HMM sufficient statistics which are ca!Culated using. 1659 -165 -.
(4) fied JNAS+Elderly acoustic models show the degrada_ tion for both the elderly test speakers and the JNAS test. Table 10: Speaker adaptation by speaker selection and HMM sufficient statistics by elderly acoustic model and. speakers. Thus, the elderly acoustic models and the usual adult models are required separately. JNAS acoustic model initial modcl is elderly acoustic model ロlOnophone. PTM. initial model is. JNAS. acoustic model. monophone. PTM. JNAS. test speaker. 78.5 86.6. I I. lnitial. I. JNAS. test speaker. initial. 83.6 92.1. I I I. elderly tcst speaker. adapted. initial. 81.9 90.6. 81.3 88.9. adapted. initial. I. I I. We tested a MLLR speaker adaptation method for the elderly speech. The adaptation by the elderly PTM model as an initial model shows 1.9% higher than JNAS. adapted. 83.7 91.2. acoustic model. This means, initial model is importa nt for MLLR, and the elderly acoustic model is useful for. clderly test speaker. 77.0 86.0. 86.6 93 8. I I I. adapted. speaker adaptation with the elderly speech. 78.7 872. We tested an unsupervised adaptation method which use the sufficient HMM statistics and speaker selection using the elderly acoustic model, the JNAS acoustic. hu Ed nu RJW白U EJW白u 白U 白羽 白羽 ao ae '' 守, ー S E Huek。u宮 。軍 回} へ 65 60. model, and the JNAS+Elderly acoustic model. As this method requires only one unsupervised utterance. It is useful for elderiy persons.. This method attains better. adaptation than MLLR by 10 utterances.. 6.. Acknowledgement. This paper reports a part of work done by a project by. NEDO(New Energy and Industrial Technology Depart ment Organization). �一一一一一一一一ー 11. ー. 7.. -+- initial model 一一+柚pted model. 21 test speaker. 31. References. [1] Stephen Anderson, Natalie Liberrr出1, Erica Berrト stein, Stephen Foster, Erin Cate, Brenda Levin, “RECOGNITION OF ELDERLY SPEECH AND. 41. VOICE-DRIV EN. DOCUMENT. RETRIE VAL",. ICASSP'99, Vol.l, pp.145-148, 1999.. Figure 2: Adaptation improvement for each speaker. [2] A.Lee, T. Kawahara, K. Takeda, K. Shikano, "A NEW PHONETI叩CT官IED-MI庇XTURE MODEL FOR EF FICIENT DECODING. the JNAS acoustic model. International Conference on Acoustics, Speech, and. Table 10 shows the word correct rates after the adap. Signal Processing, SP-Pl・8, June, 2000. tatioll with the elderly acoustic model (upp巴r) and th巴. [3] T.Kawahara,. JNAS acoustic model (lower). From the results, the el. N.Minematsu,. deriy initial model is 5.0% better than the JNAS initial. M.Yamamoto,. model for monophone, 4.0% better for PTM. The elderly. A.Yamada,. K.Takeda ,. A.Itou,. T.Utsuro,. K.Ito,. K. Shikano,. LARGE VOCABULARY CONTINUOUS SPEECH. for the elderly speakers.. RECOGNITION" ,. Illternational Conference 011 Spoken Language Processing, Ob (16)-V-07, pp.IV-. From the comparison with the adaptation results in Table 9 and Table 10, the elderly acoustic model is bet. 476-479, October,2000. ter as the initial HMM model than the JNAS+Elderly. [4] Katunobu Itou, M泳io Yamamoto, Kaz町a Takeda, Toshiyuki Takezawa, Tatsuo Matsuoka, Tetsunon Kobayashi, Kiyohiro Shikano, Shuichi Itahashi,. acoustic model for the elderly speakers. The same is true for the JNAS test speakers. for. T.Kobayashi,. S.Sagayama,. “FREE SOFTWARE TOOLKIT FOR JAPANESE. acoustic model is useful as an initial adaptation model. The improvements of word correct rates. A.Lee,. each el. “JNAS:Japanese speech corpus for large vocabulary. derly speaker are shown in Figure 2. The horizontal axis. continuous speech recognition research", The Jour nal of the Acoustical Society of Japan (E), Vo1.20,. notes test speakers who are sorted according to the word correct rates of the pre-adaptation (speaker-independent. No.3, pp.199-206, 1999. model). Figure 2 shows that the low correct speakers are. P.C.Woodland, “Maximum likeli hood linear regression for speaker adaptation of con tinuous density hidden Markov models" , Computcr. highly improved.. [5] C.J. Leggetter,. 5.. Conclusion. Speech and Language, vol.9, pp.171-185, 1995. We evaluated elderiy speaker acoustic models in LVCSR. [6] Shinichi. The elderiy speaker PTM acoustic model attains 2.9%. Yosh回wa,. Akira Baba,. Kanako Mat. sunami, Yuuichiro Mera, Miichi Yamada, Kiy ohiro Shikano, “UNSUPERVISED SPEAKER ADAPTA. better word correct rates compared with those by the usual adult PTM acoustic model. The results of gender. TION BASED ON SUFFICIENT HMM STATIS TICS OF SELECTED SPEAKERS", ICASSP,. dependent models show that it is especially di伍cult to recognize elderly male speaker speech.. 2001.. We trained the JNAS+Elderiy acoustic models from the elderiy database and the JNAS database. These uni-. 1660 -166 -.
(5)
図
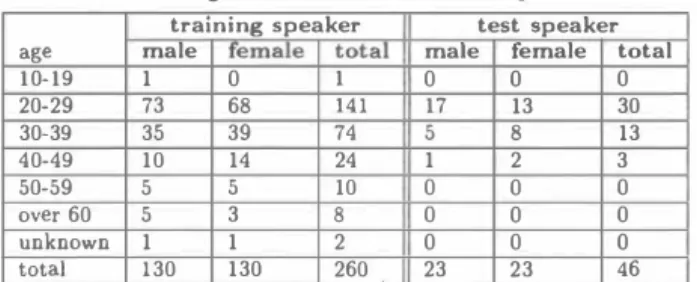



関連したドキュメント
This paper analyzes the relationship between the level of citizen participation,the degree of citizen's satisfaction with PI, and the degree of recognition of the plan, by using
Recognition process with a laser-assisted range sensor(B) 3.1 Principle of coil profile measurement This system is only appii~ble fm the case where the coils are all
This study examined the influence of obstacles with various heights positioned on the walkway of the TUG test on test performance (total time required and gait parameters)
We concluded that the false alarm rate for short term visual memory increases in the elderly, but it decreases when recognition judgments can be made based on familiarity.. Key
For other K , it appears that the Arone spectral sequences are organized more usefully than the older Anderson spectral sequence [An] for computing the homology and cohomology of Map
Summarizing, in the case in which, at the initial time, the price is below the fundamental value and the market is dominated by chartists while fundamentalists own the total wealth,
We have found that the model can account for (1) antigen recognition, (2) an innate immune response (neutrophils and macrophages), (3) an adaptive immune response (T cells), 4)
To deal with the complexity of analyzing a liquid sloshing dynamic effect in partially filled tank vehicles, the paper uses equivalent mechanical model to simulate liquid sloshing...