Theoretical Analysis of Musical Noise Generation in Noise Reduction Methods with Decision-Directed A Prioti SNR Estimator
全文
(2) In (9) and (10), we can comrnonly estimate EIIN(f, T)門 by averaging the noise power spectra in the speech absent time period, or by using other estimation methods [2], [6]. However, since we cannot 巴stlma総EIIS(f, TW] in advance, a priori SNR ç(f,T) is approximately calculated via the following the DD approach;. some unprocessed noise signals, such as an int巴rfering speech sig nal, is also high, but we do not recognize sp巴ech as musical noise. Henc巴, we tum our attention to the change in kurtosis between be fore and after signal processing to identify only the musical-noise components. Thus, we adopt the kurtosis ratio as a measure to as sess日1Usical noise [3). This measure is defined as. kurtosis rat io = kurtproc/kurtorg,. t(f,T) =α,(f,Tー1)G2(f,Tー1) + (1ーα)F [γ(I,T)一1], (11). (3). where α is a fo惇ttI 時 factor and F[.] is a 目 O∞oring fi釦伽H肌r The for屯E巴etting factor αof around 0.98, namely, 0.97...0.99, is mostly used in the past studies. Th巴se values are, however, decided experimentally, and there is no theoretical pr∞f for the justification of using such magic nurnber. One of the main p岬oses in this paper is to justify the optimal forgetting factor via theoretical analysis.. where kurtproc is the kurtosis of th巴 processed signal and kurtorg is the kurtosis of the observ巴d signal. This m巴asure increases as the amount of generated musical noise increases. In [3], it was reported that the kurtosis ratio is strongly correlated with the human p巴rcep tion of musical noise. 2.2. Analysis of amount of noise 陀duction [4]. We analyze the amount of nois巴 reduction via processing. Hereafter, we defin巴 th 巴 noise reduclion rale (NRR) as a m巴asure of出e nOlse reduction performance, which is defined as the output SNR in dB I1Ùnus th巴 input SNR in dB. The NRR is NRR =. 10l0glO(E[s�ut]/E[n�ut])/(E[s?n]/E[n?n)),. 3.THEORETICAL ANALYSIS OF NOISE REDUCTION METHODS WITH DD APPROACH. 3.1. Higher-order moment. In this s巴ction, we analyze WFωd the MMSE-STSA estimator based on the higher-order-statistics analysis. More specifically, we mathematically derive the higher-order mom巴nts of output power spectra for each method. As describ巴d in Secs. 2.1 and 2.2, the Isトorder moment is used to calculate 出巴 NRR (amount of noise reduction), and the 2nd・and 4山ーorder moments are used for getting the kurtosis ratio (amount of musical noise generation). Hereafter, random variables x and y repres巴nt the power spec tra of noise N(f, T) and its processed output Y(f,T), respectively. Signal processing in WF or the MMSE estimator with 由e DD ap proach can be interpret巴d as slochaslic variable transform from x to y, and more importantly, this is a nonlinear recursive (infinite) pr∞:ess for x because the DD approach requires infinite number of samples of the past N(f,T) (T =T O, T_), • • ., Tー∞ to output the specific Y(I, TO) at the time TO. Thus, assul1Ùng that X is indepen dent and identically distributed (i.i.dよwe can wnte出e mth-order moment of Y as. (4). where Sj n and Sout ar巴山e input and output speech signals, and njn and nout ar巴出巴 input and output noise signals, r巴spectively. If we assum巴 that the amount of noise reduction is much larger than that of speech distortion in processing, i.e., E[s�uも] c:= E[SfnL th巴n NRR. c:=. 1010g)oE[n?n]/E [ηLJ=10loE10μr/μし. (5). whereμ) is the Ist-order moment of observed signal power sp巴ctra, andμiIS th巴 1 st-order moment of processed signal power spectra 2.3. Noise 1、eduction methods with DD approach. ). In this subsection, we briefty introduceWF and the MMSE STSA es timator. We apply short-time Fourier analysis to由巳obs巴rved signal, which is a I1Ùxture of target speech and noise, to obtain出e time fr巴quency時nal X(f,T) = S(I,T) + N(I,T), where X(I,T) is the observed signal, j denotes the frequency subband, and T is the frame ind巴x. S(I,T) and N(f,T) denote the input speech and noise signals. The signal processing procedures of WF and the MMSE STSA estimator ar巴 generally formulated as. Y(f,T) = G(J, T)IX(J,T)I巴xp(jarg(X(J,T))),. μm = =. (6). and that of th巴 MMSE STSA estimator is defined as. \. ). (8). where 10 and Ir紅e the modified Bessel functions of zero and first order, and v(! ,T) =γ(j,T)ç(j,T)/(l +と(f,T)). Also, ç(l,T) andγ(1,T) are a priori and a posteriori SNRs, which are de白ned as. ç(l,T) = E[IS(I,T)12]/E[IN(I, T)12], ,(1,T) = IX(f,T)12/E[IN(f,T)12].. (9) (10). Z 刊 h 門川山m いヤ 凶 z町(付ω 仏 0 ,… x )し, ... ,X品川Xooω∞叫). a. i) 仇附州 ) (12). wh巴re山町ansformation function y - h( xo, x) ,・・・,x∞) is de自ned to represent the relation among IY(I, TOW and {I N(I,TOW, . � I!,!.c!:.. �ー∞ ) IつinWF as calculated via (6), (7) and ( ) )), or i n the MMSE STSA estimator via (6), (8) and (11). (7). (I,T) ν(I,T) GSTSA (1,T) =r(1. 5) 一一�� cxp(一 一τ.:1. ) γ(!,T) / 〆 )] [ v(!,T)) . .u _\T (v(!,T) 一.0 一 \ +', ν(1, T)Ir I 一.0 一 ) J (1+ν(f, T))lo (I :...2 - \J' • "� \ :...2. ∞. dxodx) ・・・dx∞可. where Y(I, T)】S出e 巴出初ced target speech si伊al, G(I,T) is出巴 gain function of each method. The gain function ofWF is defined as. GWF(I,T) =と(1,T)/(l +と(1,T)),. 100∞州ω 100∞ 100 100∞. '. 32N -aI叫ation 叫her-ordermoment. In this subsection, a practical calculation method of the higher-order mom巴nts is described. Since the higher-order moment (12) cannot be expressed by an analytical form, we should introduce som巴 ap pro氾mations and a nurnerical integration technique for calculating th巴 higher-order moment. First, we truncate the infinite time se qu巴nces within the past T samples, which is long enough to maint創n th巴 calculation accuracy. Next, instead of the multiple integrals, a numerical integral formula is applied in the range of Xi from 0 to!1. For example, w巴 can use the Newton-Cotes metl】od that is.
(3) a comrnonly used numerical integral forrnula with equally sarnpl巴d function values, which is given by. μm竺2二乞. kO=1 k,=1. L hm(Xko,Xk"... ,XkT)I1P(Xk;)叫い. kT=1. 包=日. (13). =. ∞ ∞ h"' ( xo," んr んf. 日. -1 ' ,X ) 日 Z7 田 p( 一 向) ∞ r(η). ∞. 2訪市主主 主1hm(zMl,,z忠)EuZl, T. ( 15). wh巴re c' is the nurnber of sample points in the integrand and ki =. x��J. are detìned as the roots of 1,2, . . . , cl The sarnpling abscissas th巴 gen巴ralized Gauss-Laguerre polynomial. LP-1I(←f(η AIso,恥W巴ゆt. γp 手 ω. {回p(ーが+ηー1 }.. (16). wtJ is calculated as. wL�J = r(ぷ + η)(山口J). -1 [五時一円xL�J)]. 。. (η= 1.0) (η=0.6). I (a). 活 30 c. i 20 0.8 0.82 0.84 0.86 0.88 0.9 0.92 0.94 0.96 0.98. 1. 0.8 0.82 0.84 0.86 0.88 0.9 0.92 0.94 0.96 0.98. 1. Forgetting factor α. 6. Forgetting factor α. Fig. 1. Theoretical behavior and experimental results for WF: (a). noise reduction rat巴, and (b) log kurtosis ratio.. (14). where r(η) is出巴 g叩una function, η is the shape pararn巴t巴r corre sponding to the type of noise, and (} is the scale pararneter of the gamrna distribution. If the input signal is Gaussian noise, th巴 p.d.f. of its power spec回ob巴ys the chi-square distribution with two de grees of fr,巴巴dom, which co汀巴sponds to the garnrna distribution with η= 1 . AIso, if出巴 input signal is super-Gaussian noise,出e p.d.f. of Its power sp巴C汀a obeys th巴 gamrna distribution with Q < 1. Th巴n, application of the garnrna distribution model to the mo ment calculation (12) 巴nables us to use g巴neralized Gauss-Lagu巴rre quadratur巴, which is a computationally eftìcient numerical integral forrnula with unequally sarnpled function values (d巴tails on general ized Gauss-Lagu巴汀e quadrature are available in [7])ー In th巴 case of noise with the shape pararneterη(the scale pararneter (} can be set to 1 without loss of generality), th巴γnth-order mom巴nts are given by. 点1. 宅ヨ. ・+-Experlme円凶value --)(--Experimen凶刈ue. 3. p(X)ニZηー1exp(-xj(})j(}ηjr(η),. 40. (η= 1.0) (η=0.6). 4. To solve the problem due to hug巴 number of MAC, we propose to introduce computationally 巴ftìcient calculation of higher-order mo ments based on出e par創netric p.d.f. model and generalized Gauss Laguerre quadrature [7]. In the following, we describ巴出巴 detail of the propos吋method and its advantage on the computational cost. First, th巴 p.d.f. of th巴 noise power sp巴C佐a is model巴d by the garnrna distribution as. ãi'. 0 一 吉 田一 的 皇豆. 3.3. Computationally efficient calculation method. モトTh回retic剖value. 5. where c is the number of sample points in the integrand, ki 1,2,・・・, C, Xk , = (ki - 1)nj(c - 1), andωki is a weight for th巴 numerical integral. The biggest problem in this calculation is a huge computational cost. For instance, T = 13 and c = 11 w巴r巴 reqUlr巴d in our prelim inary experiment to maintain th巴 calculation accuracy, resulting in C(T+1) multiply and accumulation (MAC) for the weight Wk,. This roughly co町esponds to 0.4 Peta MAC in t1oating-point arithmetic, and is obviously impossible to be processed by norrnal computers except supercomputer.. ベ)-Theoretlc剖value. -2. (17). Hereafter, we discuss the computational cost i日出巴 proposed cal culation. Generally speaking, a c'-point generalized Gauss-Laguerr巴 quadrature can yield an exact result for polynomials of up to 2c' - 1 degr巴巴s, although a c-point Newton-Cotes method does for polyno mials of up to c - 1 degrees. Thus, we can s巴t c' = cj2. This greaùy reduces the number of MAC by ( l j2T + 1)品Id in (15), compared with (13). For 巴xarnple, a use of c' = 5 in (15) r巴sults in simple 6 Giga (== 514) MAC, which can be proc巴ssed by personal computers. 4. EXPERIMENT 4.1. Experimental conditions. We calculated the NRR and kurtosis ratio using (15). The shape pararneterηof the noise p.d.f. is set to 1.0 and 0.6, and the forgetting factor αis varied from 0.8 to 0.99. The nurnber of sarnpling points in the nurnerical integral, c' in (15), is s巴t to 5, and the nurnber of frarnes coπ巴sponding to T + 1 is set to 14. Since the kurtosis of th巴 pr∞ess巴d signal changes exponentially, we depict the logari出m of the kurtosis ratio, which is referred to as the log kurrosis ratio If the log kurtosis ratio is large, it means that much musical noise generated. If the log kurtosis ratio equals zero, it means that th巴re is no musical noise generat巴d In addition, we conduct巴d a real noise r巴duction experiment in order 10 con自rm Ihe validity of our proposed theoretical analysis The NRR and log kurtosis ratio are calculated from actual noise re duction results obt創n巴d by the observed signals and processed sig nals. In th巴 evaluation experiment, the noisy observed signals were generated by adding noise signals to target speech si伊als with an SNR of 0 dB. The target speech signals were th巴 utterances of four speak巴rs (4 sentenc巴s). The length of each signal was 15 s, and each signal was sarnpled at 16 kHz. The FFT size is 1024, and the frarne shift length is 256. In these experim巴nts, we calculated the noise.
(4) ベこ〉ーTheoretlcal value (可= 1. 0) →=トTheoretical value ("司=0.) 6. ・+-Experlmen泊I value (η= 1. 0) - *-Experimental value (η=0.) 6. 0「(a) E2 宅ヨ 畠 :: 帽. 15. EE 10 呈 0.8 0.82 0.84 0.86 0.88 0.9 0.92 0.94 0.96 0.98 1 Forgetting factorα .2 帽 ー包 叫 o t. 1.6 1.2. � 0. 8 Z0 4 。. 0.8 0.82 0.84 0.86 0.88 0.9 0.92 0.94 0.96 0.98 1 Forgetting factor α. Fig .2 . Th巴oretical behavior and experimental results for MMSE. STSA estimator: (a) noise reduction rate, and (b) log kurtosis ratio.. ートWF(η= 1.0) ・0・WF(η=0.6). ...... MMSE STSA ・合-MMSESTSA. estimator(η= 1. 0) ωtimator(η=0.6). 皇.1 21 �10 58 苦6 �4. 一-./\---./\ ---./\ ー ・ λ --- ./\ … 八 一-^---/� 包 。l 八. 3. -. 0.80位0.840.86 0.880.90.920.940踊O却1 Forgetting factor α. Fig. 3. Cepstral distort】on for WF and MMSE STSA estimator.. prototype, i.e., the average of 11令(f, TW, in the tì凶10 s frames, where出e speech signal is absent. 4.2. Results. Figures I and 2 are the results of the theoretical calculations using (15) and objective evaluations for each of the noise reduction meth 吋s. We can con自rm that the theoretical values derived by our anal ysis is well consistent with thos巴 of experimental results obtained by actual noise reduction. Regarding the detailed behavior of the NRR,WF and the MMSE STSA estimator show the same tendency in that the NRR becomes higher as the larger forgetting factor αis used (see Figs. 1 (a) and 2(a)). However, regarding the log kurtosis ratio, it is of greal in teresl出at the kurtosis ratio suddenly drops aft巴r α= 0.97 in the MMSE STSA estimator (in good agreement with anoth巴r experi mental result [8]), whereas WF does nol show such a kurtosis-ratio drop. From the results, we can speculate that the kurtosis-ratio drop is the key factor of less musical noise prope口y in the MMSE STSA estimator, and we will discuss this issu巴 in the nexl subsection.. 4.3. Discussion on optimal forgetting factor. Tn this subs巴ction, we discuss the optimality on出e forgetting fac tor for each noise r巴duction method. First, we consider the MMSE STSA estimator. As shown in Fig. 2(b), the kurtosis ratio falls after α= 0.97 while higher NRRs are kept, suggesting that αshould be more closer to 1.0 from the viewpoint of less musical noise. This suggestion is, however, m.isleading becaus巴 we did not take speech distortion into account. Figure 3 shows the ceps汀al distortion for each m巴thod, when巴 we can see a monotonic increase in speech dis tortion along with larger α. ln conclusion, our theoretical analysis proves that the forgetting factor αin the MMSE STSA estimator has its sweet spot placed from 0.97 to less than 1, namely, 0.97…0.99, for achieving less musical nois巴 (kurtosis-ratio drop) as well as low spe巴ch distortion. Next, as forWF, it is also of interest that there is no kurtosis-ratio drop (see Fig. l(b)), and cons叫uentIy the specitìc αof 0.97...0.99 is meaningless in WF. Tn practice, αshould be set to more smaller value, e.g., 0.8, to avoid musical noise generation and speech distor t10n as出巴 user prefers. Thus, there is no sweet spot on出巴 forg巴ttlng factor of鴨川F. But it should be mentioned that such a meaningless valu巴 of α(= 0.97…0.99) was still us巴d confusingly in many litera tures on WF. 5. CONCLUSION. ln this study, w巴 performed a theoretical analysis of the創nount of musical noise generated in WF and the MMSE STSA estimalor based on higher-order statistics. Particularly, we calculated higher order statistics with computationally low cost and high accuracy by using generalized Gauss-Lagu巴rr巴 quadrature. From math巴matical analysis and evaluation experiments, we clari白巴d the justitìcation of using the magic numb巴r 0.98 in th巴 MMSE STSA estimator from a viewpoint of amounts of musical noise and spe巴ch distortion 6. REFERENCES. [1] P. C. Loizou, Speech enfωncement rheory and practice, CRC Press, Taylor & Francis Group FL, 2007 [2] Y. Ephraim, et a1.,“Speech enhancement using a m.inimum mean-square error sho口ーtime spectral amplitude estimator," IEEE Trans. Acoustics, Speech and Signal Processing, vo1.32, no.6, pp.1109-1121, 1984. [3] Y. Uemura, et al.,“Automatic optim.ization schem巴 of spectral subtraction bぉed on musical noise assessment via higher-order statistics," Proc.ハキ九ENC2008, 2008. [4] T. Tnou巴, et al.,“Theoretical analysis of musical noise in gen eralized sp巴C汀aI subtraction based on higher-order statistics," IEEE Trans. Audio, Speech and Language Processing, vo1.19, no.6, pp.1770-1779, 2011. [5] O. Cappé,“Elim.ination of lhe musical noise phenomenon with the Ephraim and Malah noise suppressor," IEEE Trans. Speech, and Audio Processing, vol.2, no.2, pp.345-349, 1994. [6] C. Br巴ithaupt, et al.,“Analysis of the decision-directed SNR estimator for speech enhancement with respect to low-SNR and transient conditions", IEEE Trans. Audio. Speech, and Language Processing, vol. l 9, no.2, pp.277-289, 201 1. [7] C. R. Cassity, et a1., ,‘Abscissas and coeftìcients for the gener alized Gauss-Lagu巴町巴 quadralur巴 formula using th巴 zero ordi nate," NASA Technical Memorandum, no.TMX-53099, 1964. [8] H. Yu, et a1. , ,‘A tìgure of merit for instrumental optimization of noise reduction a1gorithms," Proc. DSP in Vehicles, 2011..
(5)
図

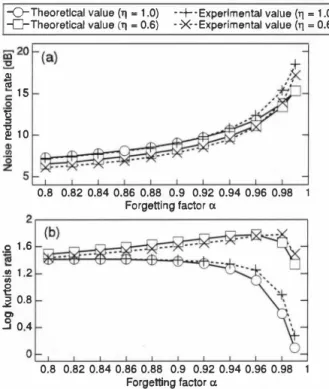
関連したドキュメント
At the same time, a new multiplicative noise removal algorithm based on fourth-order PDE model is proposed for the restoration of noisy image.. To apply the proposed model for
Using the semigroup approach for stochastic evolution equations in Banach spaces we obtain existence and uniqueness of solutions with sample paths in the space of continuous
If white noise, or a similarly irregular noise is used as input, then the solution process to a SDAE will not be a usual stochastic process, defined as a random vector at every time
In Section 3, the comparative experiments of the proposed approach with Hu moment invariance, Chong’s method is conducted in terms of image retrieval efficiency, different
In this study, it is also observed that the value of kurtosis coefficients for the independent components, which represents the noise component, can be further reduced
しかし、 平成 21 年度に東京都内の公害苦情相談窓口に寄せられた苦情は 7,165 件あり、そのうち悪臭に関する苦情は、
フロートの中に電極 と水銀が納められてい る。通常時(上記イメー ジ図の上側のように垂 直に近い状態)では、水
排水槽* 月ごとに 1 回以上 排水管・通気管* 月に 1