A tissue-conductive acoustic sensor applied in speech recognition for privacy
全文
(2) Joint sO c-EUSAI conference. Grenoble, october 2005. |ロs..出o・e・p・.Silicon I. '". 、. 100. = 〈官。 0 0 昌吉E2 [. \ デ川. 旦豆坐生旦笠巴. 1000. ・5ilicon. Figure 3: Power spectrum of the Japanese syllables Ikinil cap tured by close-talking microphone. |口Stethoac叩・・Siliconl. Figure 5目 Non-audibl巴 murmur r巴cognition using simulated noisy test data. = 。 〈,. 0 0 ZZE2 [. .. ..,・ .-12. ・Silicon. 'セ. Figure 4: Non-audible murmur recognition using clean t巴st data. 2.. Speaker-dependent non-audible murmur recognition. In this s巴ctior】, we present experimental results for speaker dependent non-audible murmur recognition using NAM mi crophones. The recognition engine was the Julius 20k vo cabulary Japanese dictation Toolkit [9]. Th巴 initial mod els were speakeトindependent, gender-independent, 3oo0-stat巴 Phonetic Tied Mixture (PTM) HMMs, trained with the JNAS database and th巴 feature vectors were of length 25 (12 MFCC, 12ð恥1FCC,ðE). The non-audible mu口nur HMMs were train巴d using a combination of supervised 128-classes regression tree MLLR [10] and MAP [11] adaptation methods. Using, how ever, MLLR and MAP combination, the parameters are prevト ously transformed using MLLR, and the transformed parame teres are us巴d as priors in MAP adaptation. [n this way, during MLLR the acoustic space is shifted and the MAP adaptation perfoηns more accurate transformations. Moreover, due to the use of a regression tree in MLLR, parameters which do not ap pear in the training data, and therefore are not transformed dur ing MAP, are transformed previously during MLLR. Due to the large di仔巴rence b巴tween the training data and the initial mod巴Is, single-iteration adaptation is not eff,巴ctlV巴 in non-audible mur mur recognition. Instead, a multi-iteration adaptation scheme was used. Th巴 initial models are adapt巴d using the training data and intermediate adapted models were train巴d. The interme diate models were used as initial models and were re-adapted using the same training data. This procedure was continu巴d un til no further improvement was obtained. Results showed, that after 5・6 iterations si伊i自C釦t improvement was achiev巴d com pared to the single-iteration adaptation. This training procedure is similar to that proposed by [12], but the object is different 2.1. 10ω. ,.∞. ,ooc. 0100'. ー邸調。. ωω. B Od B -叶 h. 旦坐里坐笠盟i.!.. 7000. Figure 6: Long-term power spectrum of offìce noise used in our expenments. small amount of data and adaptation techniques, we achieved a word performance comparable to normal-speech recognition (96.2% word accuracy). More speci白cally, using a st巴thoscope microphone we achieved an 88.9% word accuracy and using a silicon NAM microphone we achieved a 93.9% word accuracy for non-audible mu口nur recognition. The results also show the 巴仔巴ct of the multi-iteration adaptation scheme. As can be seen, with increasing numb巴r of adaptation iterations, the word accu racy was markedly increas巴d 2.2. Non-audible murmur. recognition. using. simulated. noisy test data. In this experiment, offìce noise was played back at different lev els (dBA) and recorded using a NAM microphone attached to a female talk巴r. We recorded noises at 50 dBA and 60 dBA levels The recorded noises were then superimpos巴d on 24 clean non audible murmur utterances, utter,巴d by the same female speaker, to create the simulated noisy data. The acoustic models wer巴 trained using 100 non-audible muηnur utterances recorded in a clean environment. The results showed that the performance r巴mained almost equal to that of the clean case when noise was superimposed on clean test data and recognition was performed using clean HMMs. More speci自c剖Iy, we achiev巴d 83.7%, 82.9% and 80.9% word accuracies for the clean case, the 50 dBA noise. d・ Stethoscope. 1,. 川 �. . r \ i". . , '. :'/" , ' '' 1 ';. Non-audible murmur recognition using c1ean data. .,・. /" " 主主 ,',へF _ I. .-1'1. Si川lic∞on ---. In this experiment, both training and test data were recorded in a clean environment by a male speaker using NAM micro phones. For training, 350 and for testing 48 non-audible mur mur utterances were used. Figure 4 shows the achieved results. As the figure shows, the results are very promising. Using a. H旨. s白. 出. 由. 叫. 自. 由. F由. Hl'. Figure 7・Long-term power spectrum of office noise at 70dBA level captured by NAM microphones. p. 94. - 281-.
(3) Joint sO c-EUSAI conference. Grenoble, october 2005. |ロSuperimpOB・d・Reall. [ ー 100. η←一一一一一 一一一一一一一←一一 loI-1�BA�乱 1M巾制ー. i研 j主二二. 60. 勺.G-. 40. Eヨ�. 20. 。. 制均. 01・.n. 50. 00li <<( 唱』. �. 100. No情。lev・1 [dBA]. _. i二二ニι; ',. 二 一一. 〆./. 10/ Clean. H'I.,,:,ト!,.',.ト4イトザん't.''''.',.,/,1み�1(."k今、4メ件トv 伊. ←. 一. -. -�......-._--ア� .・P .,�. τf. ふ. 'tr. 1. �\. ト. ト内i1,\rfir,\t,0,'t!1'1,H.J)'1榊トヤ片怜附寺中1柄拘洲市t;l�11引い伸一一 Figure 11: Wavefonn of cle釦vow巴1/0/ (upper) andLombard vowel/O/. 80 40 20 0. CI..n. 50. 3.. 80. Noiael・v・1 [dBA]. Figure9・Non-Audible mu口nur recogmtlOn usmg vanous types of noise. level, and the 60 dBA noise level, respectively 2.3. -.. Figure 10: Power spectrum of clean vowel /0/ andLombard vowel/O/. |口0何M・C.rロPoater口Crowd I. 80. -. --._- -一一ーー� 一. 句史M健押旬。. 60. Figure 8: Non-Audibl巴 mu口nur recognition using noisy test data (office noise). ... 】 ... o-. ........,-. Non-audible murmur recognition using real noisy test. data. In this section, we repo口 experimental results for non-audible mu口nur recognition using real noisy database. The noisy test data were recorded in an environment, where different types of noise were playing back at 50 dBA and 60 dBA levels, while a speaker was uttering the t巴st data. Four types of noise wer巴 used (office, car, poster, and crowd). For each noise and each level 24 utteranc巴s were recorded. Figure 8 shows the obtained results when using office noise in comparison with the case when the same nois巴 was supenm posed on the clean data. As can be seen, using real noisy test data, the perfonnance decreases. Namely, at the 50 dBA nois巴 level the obtain巴d word accuracy was 68.4% and at the 60 dBA noise level 47% Figure 9 shows the word accuracies for the four typ巴s of noise. The results are similar to the previous ones. With in creasing noise level, word accuracy decreases significantly. For the clean case we achieved an 83.7% word accuracy, for the 50 dBA noise 1巴vel a 66.9% word accuracy on average, and for the 60 dBA noise level a 53.3% word accuracy on average. In the case of car and crowd noises. the di仔erence between the 50 dBA and 60 dBA perfonnances is not very large. In the case of poster and office noises, the difference is larger. Although, the perfonnance using real noisy data is not markedly low and non-audible recognition is still possible, fur ther investigations are necessary. In several studies, a negative Impact e仔'ect of th巴Lombard reflex on automatic recognizers for nonnal speech has been repo口ed. It is possible, ther巴fore, that th巴 degradations in word accuracy for non-audible munnur recognition when using real noisy data, are also related to the Lombard reflex. To realize this. we also addressed theLombard reflex problem.. The role of the Lombard reDex in. non-audible murmur recognition. When speech is produced in noisy environments, speech pro duction is modifi巴d leading to the Lombard reflex. Du巴 to the reduced auditory feedback, the talker attempts to increase the intelligibility of his sp巴ech, and during this process several speech characteristics char】ge. More specificaJly, speech inten sity increases, fundamental fr巴quency (FO) and fonnants shift, vowel durations increase and the spectral tilt changes. As a re sult of these modi自cations, the p巴rfonnance of a sp巴ech r巴cog nizer decreases due to the mismatch between the training and testing conditions. To show the effect of theLombard reflex,Lombard spe巴ch is usually used, which is a clean speech utter巴d while the speaker listens to noise through headphon巴s or earphones. Even, though,Lombard speech does not contain noise compo nents, modifications in speech characteristics can be realized. Figure 10 shows the power spectrum of a nonnal-speech clean vowel/0/ and aLombard vowel/0/ recorded while listen ing to office noise through headphones at 75 dBA noise 1巴vel. The figure clearly shows the modifications leading to theLom bard reflex; power increased, formants shifted and sp巴ctral tilt chang巴d. Figure II shows the wavefonns of the clean and Lombard /0/ vowels. As can be seen, the duration and amplト tude of theLombard vowel also increased. Th巴se di仔erences in the spectra cause feature distortions (e.g., 恥1el Frequency Cepstral Coefficients (MFCC) distortions), and acoustic models trained using clean speech might fail to correctly match speech a仔巴cted by theLombard reflex. Figure 12 shows the wavefonn, spectrogram, and FO con tour of aLombard non-audible utter加ce recorded at 80 dBA As can be seen, thisLombard non-audible munnur speech has characteristics similar to those of nonnal spe巴ch. Therefore, when non-audible munnur recognition is perfonned in noisy environments, the produced non-audible mu口nur characteristics are di仔erent than those of the non-audible munnur used in the training. As a r巴sult, the perfonnance is degrad巴d, even though the NAM microphone can capture non-audible mu口nur without a high sensitivity to environmental noise. To show the e仔'ect of theLombard reflex on non-audible munnur recognition, we carri巴d out an experiment usingLom bard non-audible mu口nur test data. The data were record巴d in an anechoic room, while the speaker was listening to office noise through headphones. Since we used high-quality head-. 円〆U 6 Z J D 吋/ 円〆臼 凸 P 一.
(4) Joint sOc-EUSAI conference. i..... �_. .. Grenoble, october 2005. 100. 且 ι ...... ...... .... r._.. '..ー ' 一一ーーマ. _ 90 宰 Q) 伺. f. ;;_' J 1&; .ヰー品曲目.. 』. E '" ". -Q)Q). 0. 80. /' �� 、 1 -..- Actual scores /' ---一一一 D uration normalized scores I ---.ン 1 '-ーー ノ 70 -r 60. Figure 12: Lombard non-audible murmur r巴corded at 80 dBA. 11. 88.2%. ,. .〆. 〆. 2. 0. 4. 6. 82.3%. 10. 8. False alarms/keyword/hour. 67.3 542. h 0 •. Figure 15: Receiver Operating Characteristics (ROC) for non audible murmur keyword-spotter. 475. |ー. 0 4. :ß 80. m E c. 0 60. 60. 、、. S 40. æ 咽. 0. 、. E O Q). 、. 520. Figur巴 13: Non-Audible murmur recognition using Lombard data. -69. -60. -51. -42. 司33. -24. -15. ー6. Word insertion penalty. phones, we assumed that no noise from the headphones was add巴d to the recorded data. We recorded 24 clean utterances, 24 utterances at 50 dBA and 24 utterances at 60 dBA noise levels. The acoustic models used were trained with clean non-audible murmur data using 50 utterances and MLLR adaptation Figure 13 shows the obtained results and the e仔巴ct of the Lombard reflex on non-audible mu口nur recognition. Using clean test data, we achi巴ved a 67.39もword accuracy, using 50 dBA Lombard data a 54.2% word accuracy, and using 60 dBA Lombard data a 47.5% word accuracy. Th巴se results show an analogy between the experiments using real noisy data and the experiment using Lombard data. In both cases, the perfor mances decreased almost equally. In non-audible murmur phenomena, the Lombard reflex is also present when there is no masking noise. However, due to the very low intensity of non-audible murmur, speakers might not hear their own voice. To make their voice audible, they increase their vocal levels, and as a result, non-audible murmur. 4.. 、. Lombard印刷。h noisa I・v・I [dBA]. 、、. 50. l. 、. CI・an. 、. 。. 100. Det叫on rate -- R甲山剖e. ,. 80 � 60 � 40 ;20. Figur巴 16: Det巴ction and r句ection rates for non-audibl巴 mur mur keyword-spotter. vacy conditions. In some applications, when only a small num ber of keywords is required, a keyword-spotting system, with lower complexity and faster decoding, might be more reason able than a dictation system In a keyword-spotting approach, not only the keywords, but also the non-keyword intervals must be modeled 巴xplicitly. Our approach, was based on phonemic garbage models [1 3]. Th巴 keywords were modeled using context-d巴pendent HMMs, and monophone HMMs were used to model the non-keyword por tions. Both HMM sets were trained with non-audibl巴 murmur data recorded using a silicon NAM microphone. Fourty-thre巴 monophone HMMs were connected as to allow any sequence. The vocabulary consist巴d of 25 keywords randomly selected from JNAS database. Figure 14 shows the grammar used in our expe同ment, which allowed at most one keyword per utter ance In our experiment, the following evaluation measures were used:. A keyword-spottiog system based 00 non-audible murmur. In this section, we present a keyword-spotting experiment for non-audible murmur. A non-audible murmur-based keyword spottmg syst巴m, however, can be applied to extract a specific number of keywords from unconstrained input speech in pri-. • • •. Detection rale. The percentage of keywords detected. Rejeclion rale. The percentage of non-keywords r句ected Receiver Oper.日li昭Ch日raclerislics (ROC) and Figμre 01 Merit (FOM). The putative hits are sort巴d with resp巴ct to their scores, and the probability of detection at each false alarm is computed. The FOM is calculated as the average probability of detection between 0 and 10 false alarms per keyword. For testing, we used 18 utterances, which included one key word, and 24 utterances which did not include any keyword. Figure 15 shows the ROC curves. The figure shows, that by allowing 4 alarms per keyword we achieved 88.2% detect】on. Figur巴 14: Gramrnar used in th巴 keyword-spotter. p. 96. qu no qム.
(5) Grenoble, october 2005. Joint sO c-EUSAI conference. [7] Junqua J-C,“The Lombard Reflex and its Role on Human Listeners and Automatic Speech Recognizers", J Acollst SOC. Am., Vol. 1 pp. 510-524, 1993. rate. The achieved FO M was 85.6'1も, which is promising result The figure also shows, that using duration normalized scores the performance was d巴creas巴d. Figure 16 shows the detection and r句ection rates. To achieve higher detection and rejection rates, a word insertion penalty is tuned to d巴crease the likelihood of the garbage models. Without this tuning, however, a large num ber of false rejections (keywords are hypothesized as garbage models) appears, and as a result the detection rate decreases. Wìth word insertio日penalty tuning, we achieved a 82.5% equal rate (equal detection and r吋ection rates). 5.. [8] A. Wakao, K. Takeda, F. Itakura, '‘Variability of Lombard Effects Under Different Noise Conditions", Proceedings ojICSLP, pp. 2∞9-2012, 1996.. [9] T. Kawahara et al., “Free Software Toolkit for Japanese Large Vocabulary Continuous Speech Recognition", Pro ceedings ojICSLP, pp. IV-476-479, 2000.. [10] C. J. Leggett巴r, C. Woodland, “Maximum Likelihood Linear Regression for Speaker Adaptation of Continuous Density Hidden Markov Models", Computer Speech and Language, Vol. 9, pp. 171-185, 1995.. Conclusions. In th】s paper, w巴 presented non-audible muηnur recogmtwn ID clean and noisy noisy environments using NAM microphones. A NAM microphone is a special acoust】c device attached be hind the talker's ear, which can capture very quietly uttered speech. Non-Audible murmur recognition can be used when privacy in human-machine communication is desired. Since non-audibl巴 murmur is captured directly from the body, it is less sensitive to environm巴ntal noises. To show this, we car ried out experiments using simulated and real noisy data. Us ing simulated noisy data at 50 dBA and 60 dBA noise levels, the non-audible murmur recognition perfo口nance was almost equal to that of the clean case. Using, however, data recorded in nOlsy envlronm巴nts, the performance decreas巴d. To investigate the possible reasons for this, we studi巴d the rol巴 of the Lom bard effect in non-audible muπnur recognition and we carried out an experiment using Lombard data. The results showed that the Lombard reflex has a negativ巴 impact effect on non-audible murmur recognition. Due to the speech production modi白ca tions, the non-audible murmur characteristics under Lombard conditions are changed and show a high similarity to normal speech. Du巴 to this fact, a mismatch appears between the train ing and testing conditions and the performance decreases. As future work, we plan to investigate methods of decreasing the E仔巴ct of the Lombard reflex on non-audible murmur recog nition. A possible solution might be the adaptation of cl巴an acoustic models to several Lombard conditions. In addition to a dictation task, we also reported a keyword-spotting experiment based on non-audible murmur with very promising results. 6.. [11] C.H. Lee, C.H. Lin, and B.H. Juang, '‘A study on speaker adaptation of the parameters of continuous density hid den Markov models", lEEE transactions Signal Process ing, Vol. 39, pp. 806-814, 1991. [12] P.C. Woodland, D. Pye, M.J.F. Gàles,“Iterative Unsuper V1S巴d Adaptation Using Maximum Likelihood Lin巴arRe gression", Proceedings ojlCSLP, pp. 1133-1136, 1996. [13] R. C. Rose, D. B. Paul, “A Hidden Markov Model Based Keyword Recognition System," Proc. lCASSP, pages 129-132, 1990.. References. [1] Y. Nak句ima, H. Kashioka, K. Shikano, N. Campbell, “ Non-Audible Murmur Recognition Input Interface Using Stethoscopic Microphone Attached to the SkinぺProceed ings 01 JCASSP, pp. 708-711, 2003. [2] Y. Zheng, Z. Liu, Z. Shang, M. Sinclair, J. Droppo, L. Deng, A. Acero, Z. Huang,“ Air- and Bone-Conductive Integrated Microphones for Robust Speech Detection and Enhancement", Proceedings ojASRU, pp. 249-253, 2003 [3] M. Graciarena, H. Franco, K. Sonmez, H. Bratt,“Combin ing Standard and Throat Microphones for Robust Speech Recognition", JEEE Signal Processing Letters, Vol. 10, No 3, pp.72-74, 2003. [4] S. C. Jou, T. Schultz, Alex Weibel,“Adaptation for Soft Whisper Recognition Using a Throat Microphone", Pro ceedings ojICSLP, pp. -, 2004. [5] P. Heracleous, Y. Nakajima, A. Lee, H. Saruwatari, K Shikano,“ Non-Audibl巴 Murmur (NAM) Recognition Us ing a Stethoscopic NAM microphone", Proceedings oj ICLP, pp. 1469-1472, 2004. [6] P. Heracleous, Y.Nak勾ima, A. Lee, H. Saruwatari, K Shikano,“Audible (normal) speech and inaudible murmur recognition using NAM microphoneぺProceedings ojEU SIPCO, pp. 329-332, 2004. p. 97. Aせ 口。 つ臼.
(6)
図
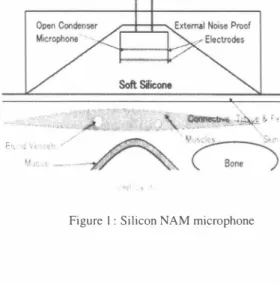
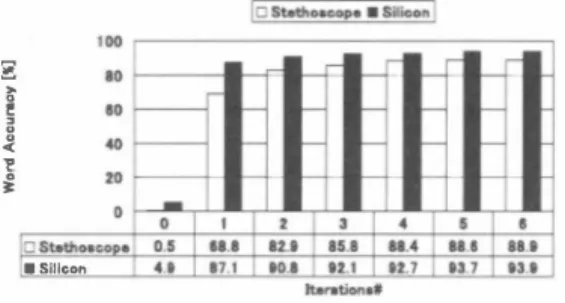

関連したドキュメント
Keywords: Convex order ; Fréchet distribution ; Median ; Mittag-Leffler distribution ; Mittag- Leffler function ; Stable distribution ; Stochastic order.. AMS MSC 2010: Primary 60E05
It is suggested by our method that most of the quadratic algebras for all St¨ ackel equivalence classes of 3D second order quantum superintegrable systems on conformally flat
Inside this class, we identify a new subclass of Liouvillian integrable systems, under suitable conditions such Liouvillian integrable systems can have at most one limit cycle, and
We present sufficient conditions for the existence of solutions to Neu- mann and periodic boundary-value problems for some class of quasilinear ordinary differential equations.. We
We have presented in this article (i) existence and uniqueness of the viscous-inviscid coupled problem with interfacial data, when suitable con- ditions are imposed on the
Then it follows immediately from a suitable version of “Hensel’s Lemma” [cf., e.g., the argument of [4], Lemma 2.1] that S may be obtained, as the notation suggests, as the m A
The proof uses a set up of Seiberg Witten theory that replaces generic metrics by the construction of a localised Euler class of an infinite dimensional bundle with a Fredholm
Section 4 contains the main results of this paper summarized in Theorem 4.1 that establishes the existence, uniqueness, and continuous dependence on initial and boundary data of a