Categorical Data Visualization and Clustering Using Subjective Factors
全文
図
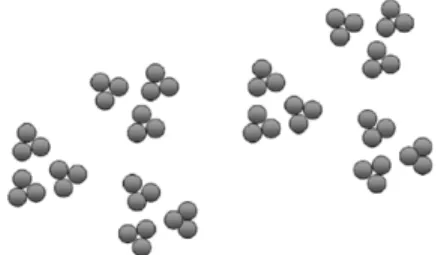
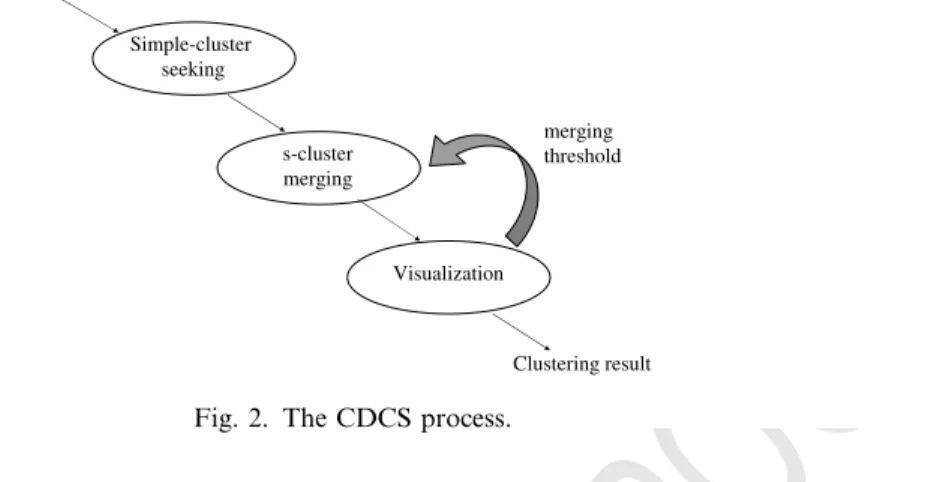


関連したドキュメント
Our work provides insight into how cluster sizes and number of subtrees in a cluster are impacted by the value of α, the maximum degree d in the tree, the relationship between c and
AHP involves three basic elements: (1) it describes a complex, multicriteria problem with objective or subjective elements as a hierarchy; (2) it estimates the relative weights
Using the results of Sections 1 and 2 one can also define in the same way as in 3.4 the set of isomorphism classes of “double” degeneration data associated with the minimal
If the interval [0, 1] can be mapped continuously onto the square [0, 1] 2 , then after partitioning [0, 1] into 2 n+m congruent subintervals and [0, 1] 2 into 2 n+m congruent
A similar structure applies to the 2 + 1 dimensional quantum Hall effect where the hierarchy of Hall plateaux can be understood in terms of an action of the modular group and
In Section 3 the extended Rapcs´ ak system with curvature condition is considered in the n-dimensional generic case, when the eigenvalues of the Jacobi curvature tensor Φ are
To address the problem of slow convergence caused by the reduced spectral gap of σ 1 2 in the Lanczos algorithm, we apply the inverse-free preconditioned Krylov subspace
In case the reader is encountering polymer models with boundaries for the first time but can appreciate an analogy with the totally asymmetric simple exclusion process (TASEP), then